Giladi, Chen
PhD student
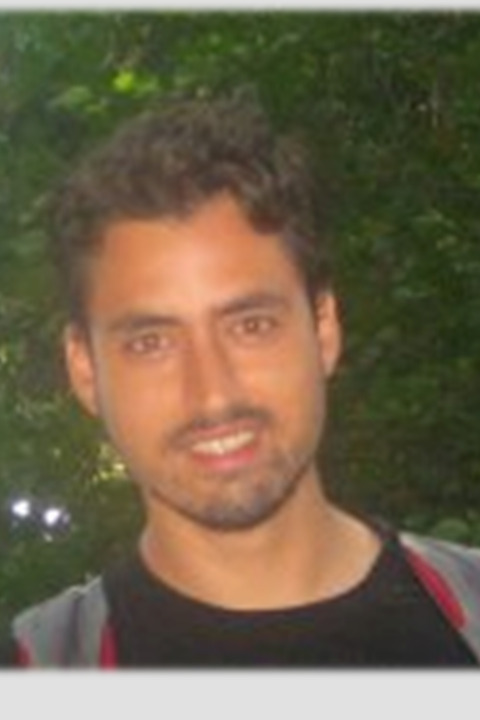
- giladic@post.bgu.ac.il
Education
-
2013-
2018,
PhD
Title is not available
with David GolombAbstract/Description: We propose to test the hypothesis that there is a logical way, which may be universal, to learn to throw and to catch flying unknown objects for the dynamical regrasping problem. Although the trajectory of a free falling body is trivial, trajectories of non-center of mass points are nonballlistic trajectories and hard to estimate. Therefore catching in flight an unknown object at a desired grasping point is difficult. We propose to solve this problem by dividing it to several steps: a. choosing the optimal throwing position, orientation and the initial translational and angular velocities in order to reach a desired intercepting point and b. accurate prediction of feasible catching configurations of the flying object by observing several examples of flying trajectories. To achieve these goals the following steps will be taken:
1. Learning to catch: Machine learning models of tossed objects will be developed. This approach will be developed with several key questions in mind: How should we track and construct a features set in order to accurately predict future position of an object? And can we recover the required feature set in unsupervised manner? The proposed mechanism will be tested experimentally by observing and tracking a set of points along the object as well as the desired gripping point. This will account for testing how the amount of tracking points and their distribution along the object affect the prediction capability of our model. Also we will investigate whether or not the proposed model is capable of predicting articulated objects interconnected by joints?
2. Learning to throw: This will involve learning the highly nonlinear mapping between the initial conditions such as: position, angular velocity, orientation etc, and the desired configuration at the intercepting point. The approach used is to first define the final configuration state, and from there to seek out what should be the initial throwing parameters, so that it will reach our desired state. This problem is ill stated since there is one to many optional solutions. To overcome this limitation we proposed two ways: back tracking algorithms and a local simulation experiment r-meshing algorithms that will be develop. -
2011-
2013,
MSc
Title is not available
with Oleg KrichevskyAbstract/Description: One of the most compelling hypotheses concerning the design principle that governs the neural code is that it increases encoding efficiency. Previous studies found that single cells perform adaptation to changes in illumination and contrast: this strengthens the hypothesis of encoding efficiency. However the studies dealing with adaptation tend to treat each ganglion cell as an independent channel of information from the eye to the brain, and thus only reflect part of the processing mechanism of the retina. Given the limited capacity of the optic nerve and the statistical dependencies between neurons, we also raise the issue of the network adaptation, and whether the network follows the same design principles for increasing encoding efficiency as a single neuron.
To address these questions we investigated the response of salamander retinal ganglion cells using a model-based approach to analyze the spike train statistics for individual and pairs of ganglion cells. Our findings show that adaption occurs in a single cell, which is consistent with previous work. Second, to reconstruct the interaction network, we used generalized linear models and maximum entropy models. This allowed us to assess the changes in coupling filters predicted to be due to adaption effects of each neuron in the network.
Our results indicate plasticity in retinal processing in which the interactions between cells change due to adaptation. Furthermore, interactions between cells improve the network ability to adapt more effectively in order to increase the efficiency of visual encoding. The evidences suggest that the retina chooses the coupling filter that minimizes the redundancy between two neurons, thus revealing a design principle of the early visual system.